In the last few years, we've watched AI sweep across industries like finance, healthcare, manufacturing, and transportation with breathtaking speed. Yet, e-commerce—arguably one of the most data-rich and fast-moving sectors—has lagged behind. For an industry that thrives on efficiency, why has e-commerce not fully embraced AI and automation?
Let’s explore the gap, understand the friction, and envision what the future could look like if e-commerce finally catches up.
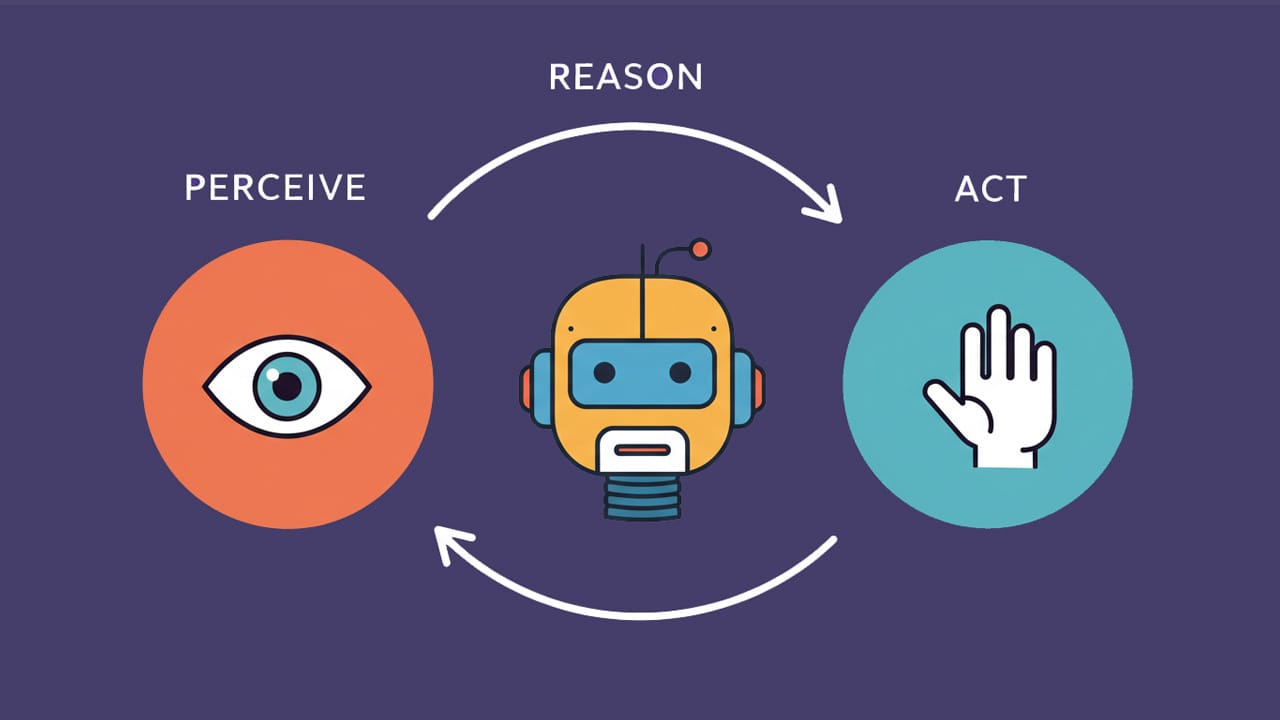
Industries Surging Ahead with AI
AI in healthcare is revolutionizing diagnosis. IBM Watson Health, for instance, helps clinicians make faster, more accurate decisions. In finance, JPMorgan Chase uses AI to review documents—what used to take 360,000 hours annually is now completed in seconds. The global AI in finance market alone was valued at $9.45 billion in 2021 and is projected to reach $247 billion by 2030.
Manufacturing, too, is in the midst of an AI renaissance. Predictive maintenance, robotics, and supply chain automation have reduced downtime by up to 20% and improved production efficiency. Even agriculture—an industry not typically associated with cutting-edge tech—has embraced AI through smart irrigation, crop monitoring, and autonomous tractors.
So why hasn’t e-commerce, built on real-time data and customer behavior, made the same leap?
The E-commerce Paradox: Digitally Native, Yet Manual
It’s not that AI isn’t used in e-commerce—it is. But it’s largely concentrated in a few visible areas: product recommendations, dynamic pricing, and basic chatbots. Under the hood, however, much of e-commerce is still surprisingly manual:
Product onboarding is often done by humans, one SKU at a time.
Vendor approvals, return reconciliations, and catalog updates involve spreadsheets and emails.
Customer service, despite automation tools, is still heavily reliant on human agents.
Analytics dashboards exist, but insights are often delayed, not real-time, and rarely predictive.
Compare that to an AI-assisted manufacturing line where machine vision spots defects instantly or a bank where AI flags fraud in milliseconds.
Why Is E-commerce Lagging?
Several intertwined reasons explain this paradox.
1. Fragmented Tech Stacks
E-commerce businesses use a mix of Shopify, ERP systems, CRMs, Google Analytics, Meta Ads, logistics APIs, and more. Stitching these systems together for end-to-end automation is difficult, especially for small and mid-sized brands. Unlike a hospital with an integrated EMR or a bank with centralized data infrastructure, e-commerce stacks are often cobbled together.
2. Incentive Misalignment
For marketplaces like Amazon or Flipkart, the incentives are often focused on maximizing ad revenue and conversions. Automation that helps sellers might not align with platform priorities. For sellers, immediate sales take priority over long-term automation investments.
3. Lack of AI Fluency
AI adoption requires not just tools but understanding. Many e-commerce founders are design- or product-first, not data-native. Hiring or outsourcing AI talent is expensive and often not ROI-positive in the short term, especially when margins are razor thin.
4. Data Quality Problems
AI thrives on clean, labeled, and abundant data. E-commerce data is messy: product names vary, attributes are inconsistent, returns are logged differently across systems. Until foundational data problems are fixed, AI’s effectiveness remains limited.
5. Too Many Tools, Not Enough Platforms
There are hundreds of tools for reviews, inventory, returns, personalization—but very few unified automation platforms. The abundance of niche tools leads to integration fatigue and limited visibility, making AI feel more like a gimmick than infrastructure.
What’s Possible with True E-commerce Automation?
The possibilities are vast. Imagine:
Product Research Bots: Agents that analyze Amazon, Shopify, and global search trends to suggest your next winning SKU.
Zero-Touch Customer Service: LLM-powered agents resolving over 90% of queries, across languages, tone-adapted, and context-aware.
AI Merchandising: Auto-generated product titles, descriptions, meta tags, and lifestyle imagery based on just a sample photo.
Return Prediction and Prevention: Models that flag likely-returned items before shipping, nudging users with size fit tips or better alternatives.
Revenue Intelligence: Not just dashboards, but agents that recommend ad spend changes, discount timings, and stock reorders in natural language.
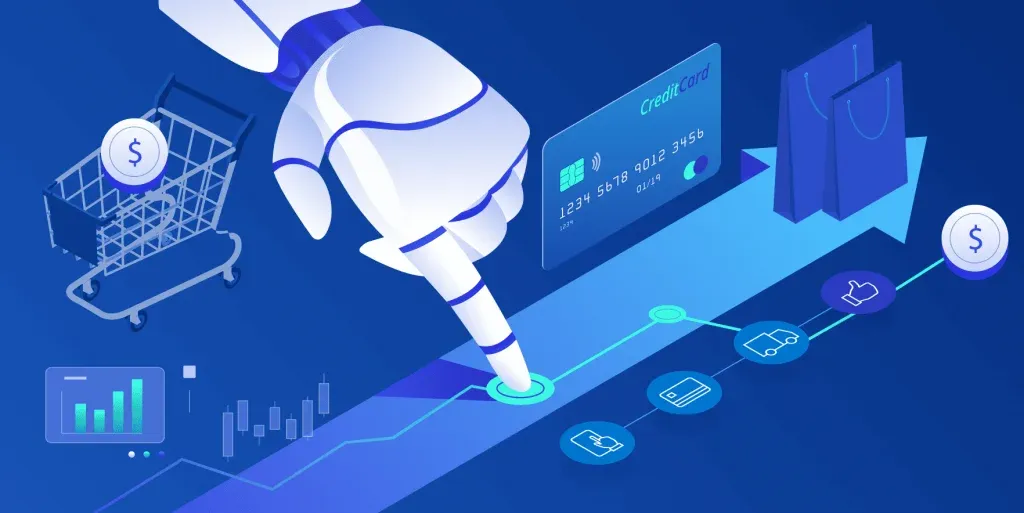
The Opportunity is Massive
McKinsey estimates that AI could deliver up to $1.4 trillion annually in retail and CPG value. Yet, most of this remains untapped. While large retailers like Walmart and Amazon are building in-house AI teams, the long tail of D2C brands and small sellers still rely on manual grunt work.
This gap is an opportunity.
Much like Stripe and Shopify abstracted payments and storefronts for millions of merchants, we’re seeing the rise of AI-native platforms that abstract catalog enrichment, competitive analysis, customer support, and more.
The Tipping Point is Near
As open-source LLMs become more efficient and data integration tools mature (think: Airbyte, ClickHouse, LangChain), the friction to adopt AI in e-commerce is dropping. The next 24 months will likely see a surge in purpose-built AI co-pilots and agents for e-commerce.
What used to take a team of VAs and a week can now be done by an agent in minutes: creating a 100-product catalog, generating a month’s worth of SEO blogs, identifying hot-selling competitors, or reconciling refunds with orders.
Closing Thoughts
E-commerce doesn’t suffer from a lack of AI potential—it suffers from fragmentation, legacy processes, and a short-term mindset. But change is coming. As AI becomes more plug-and-play, e-commerce automation will no longer be a luxury—it will be the baseline.
The brands that embrace this shift early will outpace competitors not just in speed but in margin, quality, and innovation. The future of e-commerce isn’t just faster checkout or better recommendations. It’s autonomous businesses that can scale like software.
And that future isn’t far away.